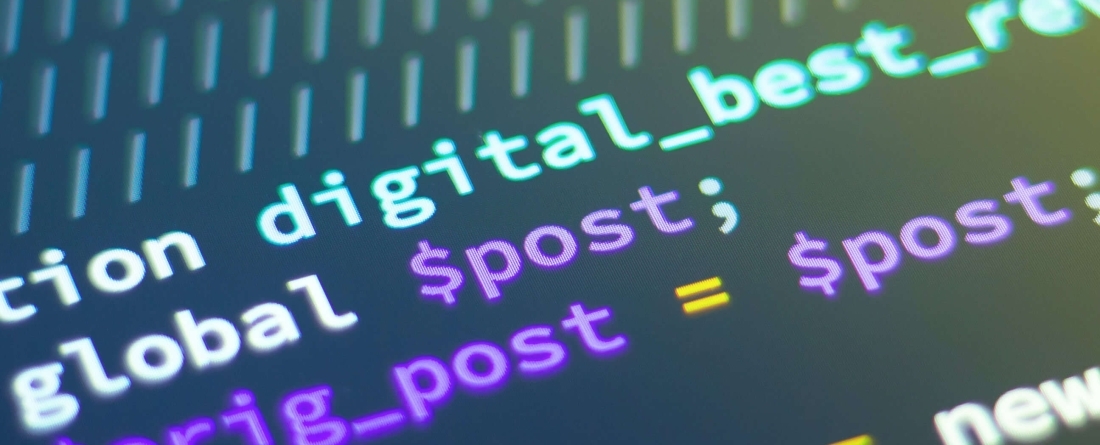
The Cyber Events Database collects publicly available information on cyber events, beginning in 2014 to the present day. It was created to address a lack of consistent, well-structured data necessary for making strategic decisions about how to invest resources to prevent and respond to cyber events.
We are currently transitioning the database onto our main website. Please register for access to the database by hitting the button below. After registering and submitting your response, follow the link displayed to receive a download of the database file.
NEW VARIABLES! The dataset now includes targeted U.S. state and county variables and dummy variables for targeted country membership in various international organizations.
The Cyber Events Database has been leveraged by:
Cyber Events Data Descriptive Statistics, 2014-2023
All graphs below come from 13,327 cyber events recorded between 2014-2023. Our full dataset, which we update on a monthly basis, currently provides records of cyber events from 2014 - June 2024.
- Exploitative attacks (or those looking to steal information ) remain the most common though disruptive attacks (aimed at interfering with a target's operations) saw a significant spike in 2022 following Russia's invasion of Ukraine before settling back to historical levels in 2023.
- Mixed effect events are also gradually increasing as more criminal actors use techniques such as double extortion, which involves data exfiltration (exploitative) and encryption (disruptive).
Publications Carousel
The increasing scale and impacts of cyber events remain an enduring concern, yet information covering the range of threat actors, motive, industry, or classified impact are scarce, fractured, or are only available through private organizations at a significant cost.
As the private and public sectors grapple with the multi-faceted problem of cyber security, they lack basic tools needed to make strategic decisions about prevention and response. Software solutions, organizational resilience, employee education, and improved system controls are among the many available options to enhance cybersecurity. Yet, it is difficult to make strategic decisions about how to invest scarce resources without an understanding of what types of cyber threats are most common in a specific industry or critical infrastructure sector and what their effects might be.
There exists a number of smaller niche repositories, news sites, and blogs that catalog cyber events, yet the data is often not well structured or consistently coded. To address this gap, CISSM and GoTech have launched the Cyber Events Database project with information on cyber attacks from 2014 to the present. The Cyber Events Database allows users to distill analytical insights on cyber threats to specific industries and regions, trends over time, and the behavior of different threat actors.
The dataset contains structured information across several categories and is now available to researchers and industry partners. The Cyber Events Database utilizes automated techniques paired with manual review and classification by researchers to acquire and structure data from a variety of open news sites, blogs, and other specialty sites that identify and discuss publicly attributed attacks. The data is updated monthly and yields information about the threat actor, motive, victim, industry, and end effects of the attack.
CISSM and GoTech have made descriptive information freely available to the public. Researchers or public officials interested in the detailed records or access to the dataset in its entirety should contact Dr. Charles Harry at charry@umd.edu
Researchers who plan on using the data for publication should cite the following: Harry, C., & Gallagher, N. (2018). Classifying Cyber Events. Journal of Information Warfare, 17(3), 17-31.
Data is collected using a mixed-methods approach that leverages a Python application to “scrape” data from relevant cyber sources which is then reviewed and coded by the research team to (1) ensure the events identified meet the definition of a cyber event, (2) consistently categorize threat actor type, motive, threat actor country, and targeted country, and (3) accurately classify the industry and specific effects the event achieved. Events are categorized based on the effects they produced according to a structured taxonomy.[1] Attributions are taken from the source material. We do not conduct our own analysis to validate that assessment.
To gather candidate material, we employ the use of a customized Python script that query a list of known websites on the open internet and dark web, each of which link to individual entries, articles, and/or subpages that are candidates for inclusion. Using site-specific algorithms, the script accesses each site’s main landing page via a predetermined URL and returns data (date published, title, URL, and article preview) in HTML format for processing by researchers (Figure 1). The source combines this information with the local date/time of the page being accessed, as well as the title associated with the overarching website. All of this information is included in a single row of two comma-separated values (.csv) files that are added to the user’s machine by the script.
The Python script makes use of the csv, datetime, and urllib internal Python libraries, as well as Beautiful Soup 4, an external library that facilitates the bulk of the data extraction. At this stage in development, the script is fully functional via an interpreter or development environment that runs Python 3.8.
The script makes no effort to determine suitability of the candidate cyber event. All linked entries/articles are included in a daily deduplicated file to be reviewed by a researcher, who makes final judgements as to whether events are valid members of the dataset. We define a cyber event as the end result of any single unauthorized effort, or the culmination of many such technical actions, that engineers, through use of computer technology and networks, a desired primary effect on a target. The dataset chiefly records individual cyber events where a discernible effect was achieved by the threat actor (e.g. hacker). To be included in the dataset, each event must be traced back to an underlying source describing details surrounding the event itself.
- Event Date (event_date) – Date or estimated date that the event occurred in DD-MM-YYYY format. Estimated dates are accurate to the month and are indicated as the first day of that month
- Year (year) – Year event occurred in YYYY format.
- Actor (actor) – String variable indicating the name of the organization or individual responsible for the event; “undetermined” if unknown.
- Actor Type (actor_type) – Categorical variable indicating the nature of the actor responsible for the event:
- Criminal – Organization that illicitly accesses networks for financial gain.
- Nation-State – A government agency, military, or affiliate thereof
- Terrorist – a non-state actor seeking to influence political or military conditions by targeting civilians
- Hacktivist – an individual or group motivated by social or political activism
- Hobbyist – an individual motivated by curiosity or prestige
- Organization (organization) – String variable indicating the name of the target organization whose networks were illicitly breached
- North American Industry Classification System (NAICS) Code (industry_code) – Two-digit NAICS code defining the target organization.
- Industry Name (industry) – String variable indicating the name of the NAICS code category
- Motive (motive) – Categorical variable indicating the intended results sought by the actor committing the event
- Protest – The disruption of services in order to send a political or social message to the target organization, or to a government or population indirectly.
- Sabotage – The intentional, irreparable destruction of information, networks, or devices
- Espionage – Accessing of networks for the purposes of intelligence or surveillance
- Financial – Exfiltrating sensitive data for direct or indirect financial gain.
- Event Type (event_type) – Categorical variable indicating whether the primary end effects of the event were disruptive, exploitative, or a mixture of the two.
- Disruptive – Impedes the target organization’s normal operations
- Exploitive – Illicitly access or exfiltrate sensitive information such as personal identifiable information, classified information, or financial data.
- Mixed – Event incorporates both disruptive and exploitative elements, such as a ransomeware attack.
- Event Sub-type (event_subtype) – Categorical variable further classifying the nature of an event based on the part of the target organization’s IT infrastructure that was most seriously impacted, regardless of the tactics or techniques used to achieve the final result.
- Disruptive events:
- Message Manipulation – Interference with the target organization’s ability to accurately present or communicate information to its customer base, constituency, or other audience.
- Examples: These attacks include the hijacking of social media accounts, such as Facebook or Twitter, or defacing a company website by replacing the legitimate site with pages supporting a political cause.
- External Denial of Services – Executed from devices outside of the target organization’s network to degrade or deny its ability to communicate with other systems.
- Examples: Many types of Distributed Denial of Service (DDoS) attacks would fit into this category, including ICMP flood, SYN flood, or ping of death. A Border Gateway Protocol (BGP) hijack that diverted Internet traffic away from a targeted organization’s website would also fit in this category.
- Internal Denial of Services – Executed from inside a target organization’s network to degrade or deny access to other parts of the IT network.
- Examples: An attacker who gained remote access could move laterally inside an organization’s network to reset a core router to factory settings, preventing devices inside the network from communicating with each other. They could also install malware on a file server and disrupt data sent to and received from user workstations.
- Data Attack – The manipulation, destruction, or encryption of data in a target organization’s network.
- Examples: Common techniques include the use of wiper viruses and ransomware. Using stolen administrative credentials to manipulate data and violate its integrity, such as changing grades in a university registrar’s database, would fall into this category, as well.
- Physical Attack – The use of IT components, such as SCADA systems, to manipulate, degrade, or destroy physical systems.
- Examples: Current techniques used to achieve this type of effect include the manipulation of Programmable Logic Controllers (PLC) to open or close electrical breakers, leading to a de-energizing of that portion of the grid, or the utilization of user passwords to change settings in a human machine interface so that a blast furnace overheats and is destroyed.
- Message Manipulation – Interference with the target organization’s ability to accurately present or communicate information to its customer base, constituency, or other audience.
- Exploitive events – exploitative events are classified by the part of the target organization’s IT infrastructure from which the malicious actor steals the information.
- Exploitation of Sensors – The theft of data from a peripheral device, such as a credit card reader, smart TV, or baby monitor.
- Example: In 2013, the Target corporation had thousands of their Point of Sale (PoS) devices compromised, leading to the loss of over 40 million customer credit card numbers.
- Exploitation of End Host – The theft of data stored on user’s desktop computers, laptops, or mobile devices.
- Examples: Common tactics currently used include sending a malicious link for a user to click or leveraging compromised user credentials to log in to an account.
- Exploitation of Network Infrastructure – The theft of data through direct access to networking equipment such as routers, switches, and modems.
- Example – In 2018, over 500,000 routers worldwide were infected with VPNFilter malware which maintained access to devices through the compromise of user credentials and left open the potential for information to be hijacked.
- Exploitation of Application Server – The use of a misconfiguration or vulnerability to gain access to data in a server-side application (e.g. a database) or on the server itself.
- Examples: The hacker in the 2015 Office of Personnel Management data breach used a SQL injection to access millions of records with sensitive information about current and former government employees. This category also includes the theft of data from Sony Pictures achieved when the hacker gained direct access to an e-mail server with organizational correspondence.
- Exploitation of Data in Transit – The acquisition of data moving between devices.
- Example: Unencrypted data might be acquired as it is sent from a PoS device like a credit card reader to a database, or when somebody makes a purchase over the Internet from their laptop through an unsecured wireless hotspot at a local coffee shop.
- Exploitation of Sensors – The theft of data from a peripheral device, such as a credit card reader, smart TV, or baby monitor.
- Disruptive events:
- Event Description (description) – String variable consisting of 1-3 sentences detailing the event.
- Source URL (source_url) – String variable consisting of the URL from which the data was pulled
- Target Country (targeted_country) – String variable consisting of 3-letter ISO country code for the target organization’s location.
- Actor Country (actor_country) – String variable consisting of 3-letter ISO country code for the actor’s location.
For more information about the Cyber Events Database, please contact Dr. Charles Harry at charry@umd.edu